SBU’s Markus Seeliger part of team exploring cancer drug resistance
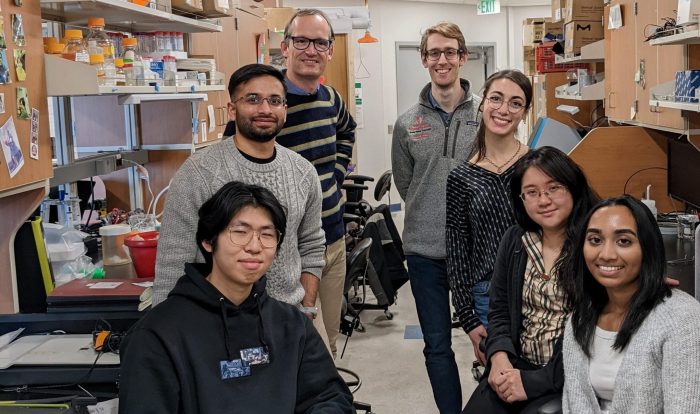
By Daniel Dunaief
When a dart hits the center of a target, the contestant often gets excited and adds points to a score. But what if that well-placed dart slipped off the board before someone could count the points, rendering such an accurate throw ineffective?
With some cases of cancer treatments, that’s what may be happening, particularly when a disease develops a mutation that causes a relapse. Indeed, people who have chronic myeloid leukemia typically receive a treatment called Imatinib, or Gleevac.
The drug works, hitting a target called a kinase, which this white blood cell cancer needs to cause its cells to continue to divide uncontrollably. Patients, however, develop a mutation called N368S, which reduces the effectiveness of the drug.
While mutations typically make it more difficult for a drug to bind to its target, that’s not what’s happening with this specific mutation. Like the dart hitting the center of a board, the drug continues to reach its target.
Instead, in a model of drug resistance several scientists have developed, the mutation causes the drug to decouple.
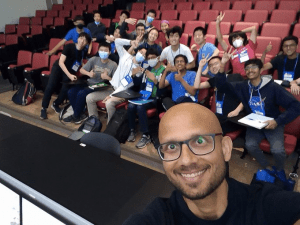
A team of experimental and computational researchers including Markus Seeliger, Associate Professor of Pharmacological Sciences at Stony Brook University, and Pratyush Tiwary, Associate Professor in the Department of Chemistry & Biochemistry at the University of Maryland, published two research papers explaining a process that may also affect the way mutations enable resistance to other drugs.
Seeliger described how different disease-associated mutations bind to Gleevac in a paper published in the Proceedings of the National Academy of Sciences.
Working with scientists at Memorial Sloan Kettering Cancer Center and Goethe University in Frankfurt, Germany, Seeliger used nuclear magnetic resonance spectroscopy, or NMR. The researchers showed how the drug bound to its target and then released.
Understanding the way diseases like cancer develop such resistance could affect drug discovery, giving pharmaceutical companies another way to prepare for changes diseases make that reduce the effectiveness of treatments.
A ‘hot paper’
Tiwary published research in which Seeliger was a coauthor in late April in the journal Angewandte Chemie that the publication labeled a “hot paper” for its implications in the field. Tiwary developed a way to simulate the kinetic processes that enable the mutated kinases to release the drug.
Tiwary created an artificial intelligence model that extended the time he analyzed the drug-protein interaction from milliseconds all the way out to thousands of seconds.
“Even within the simulation world, if you can quantitatively predict a binding affinity, that’s amazing,” Seeliger said. “It’s extremely hard to calculate kinetics, and he got that right.”
Tiwary, who started talking with Seeliger about five years ago and has been actively collaborating for about three years, uses experimental data to inform the dynamics that affect his simulations.
Seeliger “had done the experiments of the dissociation rates beforehand, but did not have a way to explain why they were what they were,” Tiwary explained in an email. “Our simulations gave him insights into why this was the case and … insight into how to think about drugs that might dissociate further.”
Drug discovery
Tiwary hopes the work enables researchers to look at structural and kinetic intermediates in reactions, which could provide clues about drug design and delivery. While he worked with a single mutation, he said he could conduct such an analysis on alterations that affect drug interactions in other diseases.
He wrote that the computations, while expensive, were not prohibitive. He used the equivalent of 16 independent 64 CPUs for one to two weeks. He suggested that computing advances could cut this down by a factor of 10, which would enable the exploration of different mutations.
“The methods are now so easy to automate that we could run many, many simulations in parallel,” Tiwary explained. Machine learning makes the automation possible.
Given what he’s learned, Tiwary hopes to contribute to future drug begin that addresses mutation or resistance to treatment in other cancers. He also plans to continue to work with Seeliger to address other questions.
Next steps
Seeliger said he plans to extend this work beyond the realm of this specific type of cancer.
He will explore “how common these kinetic mutations are in other systems, other diseases and other kinases,” Seeliger said.
He would also like to understand whether other proteins in the cell help with the release of drugs or, alternatively, prevent the release of drugs from their target. The cell could have “other accessory proteins that help kick out the drug from the receptor,” Seeliger said.
The concept of drug resistance time comes from infectious disease, where microbes develop numerous mutations.
Seeliger, who is originally from Hanover, Germany, said he enjoys seeing details in any scene, even outside work, that others might not notice.
He described how he was driving with postdoctoral fellows in Colorado when he spotted a moose. While the group stopped to take a picture, he noticed that the moose had an ear tag, which is something others didn’t immediately notice.
As for the research collaboration, Seeliger is pleased with the findings and the potential of the ongoing collaboration between experimental and computational biologists.
“The computational paper, aside from using interesting new methodology, describes why things are happening the way they are on a molecular level,” he said.