CSHL’s Peter Koo tackles deep learning
By Daniel Dunaief
We built a process that works, but we don’t know why. That’s what one of the newest additions to Cold Spring Harbor Laboratory hopes to find out.
Researchers have applied artificial intelligence in many areas in biology and health care. These systems are making useful predictions for the tasks they are trained to perform. Artificial intelligence, however, is mostly a hands-off process. After these systems receive training for a particular task, they learn patterns on their own that help them make predictions.
How these machines learn, however, has become as much of a black box as the human brains that created these learning programs in the first place. Deep learning is a way to build hierarchical representations of data, explained Peter Koo, an assistant professor at the Simons Center for Quantitative Biology at CSHL, who studies the way each layer transforms data and the next layer builds upon this in a hierarchical manner.
Koo, who earned his doctorate at Yale University and performed his postdoctoral research at Harvard University, would like to understand exactly what the machines we created are learning and how they are coming up with their conclusions.
“We don’t understand why [these artificial intelligence programs] are making their predictions,” Koo said. “My postdoctoral research and future research will continue this line of work.”
Koo is not only interested in applying deep learning to biological problems to do better, but he’s also hoping to extract out what knowledge these machines learn from the data sets to understand why they are performing better than some of the traditional methods.
“How do we guide black box models to learn biologically meaningful” information? he asked. “If you have a data set and you have a predictive model that predicts the data well, you assume it must have learned something biologically meaningful,” he suggested. “It turns out, that’s not always the case.”
Deep learning can pick up other trends or links in the data that might not be biologically meaningful. In a simplistic example, an artificial intelligence weather system that tracked rain patterns during the spring might conclude, after seven rainy Tuesdays, that it rains on Tuesdays, even if the day of the week and the rain don’t have a causative link.
“If the model is trained with limited data that is not representative, it can easily learn patterns that are correlative in the training data,” Koo said. He tries to combat this in practice by holding out some data, which is called validating data. Scientists use it to evaluate how well the model generalizes to new data.
Koo plans to collaborate with numerous biologists at Cold Spring Harbor Laboratory, as well as other quantitative biologists, like assistant professors Justin Kenney and David McCandlish.
In an email, Kenney explained that the Simons Center is “very interested in moving into this area, which is starting to have a major impact on biology just as it has in the technology industry.”
The quantitative team is interested in high-throughput data sets that link sequence to function, which includes assays for protein binding, gene expression, protein function and a host of others. Koo plans to take a “top down” approach to interpret what the models have learned. The benefit of this perspective is that it doesn’t set any biases in the models.
Deep learning, Koo suggested, is a rebranding of artificial neural networks. Researchers create a network of simple computational units and collectively they become a powerful tool to approximate functions.
A physicist by training, Koo taught himself his expertise in deep learning, Kenney wrote in an email. “He thinks far more deeply about problems than I suspect most researchers in this area do,” he wrote. Kenney is moving in this area himself as well, because he sees a close connection between the problem of how artificial intelligence algorithms learn to do things and how biological systems mechanistically work.
While plenty of researchers are engaged in the field of artificial intelligence, interpretable deep learning, which is where Koo has decided to make his mark, is a considerably smaller field.
“People don’t trust it yet,” Koo said. “They are black box models and people don’t understand the inner workings of them.” These systems learn some way to relate input function to output predictions, but scientists don’t know what function they have learned.
Koo chose to come to Cold Spring Harbor Laboratory in part because he was impressed with the questions and discussions during the interview process.
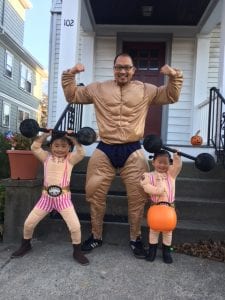
He started his research career in experimental physics. As an undergraduate, he worked in a condensed matter lab of John Clarke at the University of California at Berkeley. He transitioned to genomics, in part because he saw a huge revolution in next-generation sequencing. He hopes to leverage what he has learned to make an impact toward precision medicine.
Biological researchers were sequencing all kinds of cancers and were trying to make an impact toward precision medicine. “To me, that’s a big draw,” Koo said, “to make contributions here.”
A resident of Jericho, Koo lives with his wife, Soohyun Cho, and their 6-year-old daughter Evie and their 4-year old-daughter Yeonu.
Born and raised in the Los Angeles area, he joined the Army Reserves after high school, attended community college and then transferred to UC Berkeley to get his bachelor’s degree in physics.
As for his decision to join Cold Spring Harbor Laboratory, Koo said he is excited with the opportunity to combine his approach to his work with the depth of research in other areas.
“Cold Spring Harbor Laboratory is one of those amazing places for biological research,” Koo said. “What brought me here is the quantitative biology program. It’s a pretty new program” that has “incredibly deep thinkers.”