By Daniel Dunaief
Now what? It’s a question that affects everyone from the quarterback who wins the Super Bowl — who often says something about visiting a Disney facility — to the student who earns a college degree, to the researcher who has published a paper sharing results with the scientific community.
For some, the path forward is akin to following footsteps in the snow, moving ever closer to a destination for which a path is clear. For others, particularly those developing new technology, looking to unlock mysteries, the path is more like trudging through unfamiliar terrain.
The technology at facilities like Brookhaven National Laboratory, which includes the powerful National Synchrotron Light Source II and the Center for Functional Nanomaterials, among others, enables scientists to see processes at incredibly fine scales.
While these sites offer the promise of providing a greater ability to address questions such as what causes some batteries to die sooner than others, they also cost considerable money to use, putting pressure on researchers to ask the most fruitful question or pursue research that has the greatest chance for success.
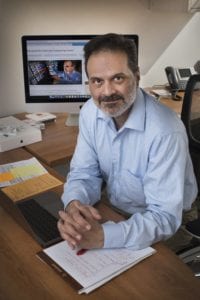
That’s where people like Francis Alexander, the deputy director of Brookhaven National Laboratory’s Computational Science Initiative, and his team at BNL can add considerable value. Alexander takes what researchers have discovered, couples it with other knowledge, and helps guide his fellow laboratory scientists to the next steps in their work — even if he, himself, isn’t conducting these experiments.
“Given our theoretical understanding of what’s going on, as imperfect as that may be, we take that understanding — the theory plus the experimental data — and determine what experiments we should do next,” Alexander said. “That will get us to our goal more quickly with limited resources.”
This approach offers a mutually reinforcing feedback loop between discoveries and interpretations of those discoveries, helping researchers appreciate what their results might show, while directing them toward the next best experiment.
The experiments, in turn, can either reinforce the theory or can challenge previous ideas or results, forcing theoreticians like Alexander to use that data to reconstruct models that take a wide range of information into account.
Alexander is hoping to begin a project in which he works on developing products with specific properties. He plans to apply his knowledge of theoretical physics to polymers that will separate or grow into different structures. “We want to grow a structure with a [particular] function” that has specific properties, he said.
This work is in the early stages in which the first goal is to find the linkage between what is known about some materials and what scientists can extrapolate based on the available experiments and data.
Alexander said the aerospace industry has “models of everything they do.” They run “complex computer simulations [because] they want to know how they’d design something and which design to carry out.”
Alexander is currently the head of a co-design center, ExaLearn, that focuses on exascale, machine-learning technologies. The center is the sixth through the Exascale Computing Project. Growth in the amount of data and computational power is rapidly changing the world of machine learning and artificial intelligence. The applications for this type of technology range from computational and experimental science to engineering and the complex systems that support them.
Ultimately, the exascale project hopes to create a scalable and sustainable software framework for machine learning that links applied math and computer science communities to create designs for learning.
Alexander “brings to machine learning a strong background in science that is often lacking in the field,” Edward Dougherty, a distinguished professor in the Department of Electrical and Computer Engineering at Texas A&M, wrote in an email. He is an “excellent choice to lead the exascale machine learning effort at Brookhaven.”
Alexander is eager to lead an attempt he suggested would advance scientific and national security work at the Department of Energy. “There are eight national laboratories involved and all the labs are on an equal level,” he said.
One of the goals of the exascale computing project is to build machines capable of 10 to the 18th operations per second. “There’s this enormous investment of DOE” in this project, Alexander said.
Once the project is completely operational, Alexander expects that this work will take about 30 percent of his time. About 20 percent of the time, he’ll spend on other projects, which leaves him with about half of his workweek dedicated to management.
The deputy director recognizes that he will be coordinating an effort that involves numerous scientists accustomed to setting their own agenda.
Dougherty suggested that Alexander’s connections would help ensure his success, adding that he has “established a strong network of contacts in important application areas such as health care and materials.
The national laboratories are akin to players in a professional sporting league. They compete against each other regularly, bidding for projects and working to be the first to make a new discovery. Extending the sports metaphor, members of these labs often collaborate on broad projects, like players on an all-star team competing against similar teams from other nations or continents.
Alexander grew up in Ohio and wound up working at Los Alamos National Laboratory in New Mexico for over 20 years. He came to BNL in 2017 because he felt he “had the opportunity to build something almost from the ground up.” The program he had been leading at Los Alamos was large and well developed, even as it was still growing.
The experimental scientists at BNL have been receptive to working with Alexander, which has helped him achieve some of his early goals.
Ultimately, Alexander hopes his work increases the efficiency of numerous basic and applied science efforts. He hopes to help experimental scientists understand “what technologies we should develop that will be feasible” and “what technologies would be most useful to carry experiments out.”